Full PDFs free on GitHub. To support us, visit Patreon.
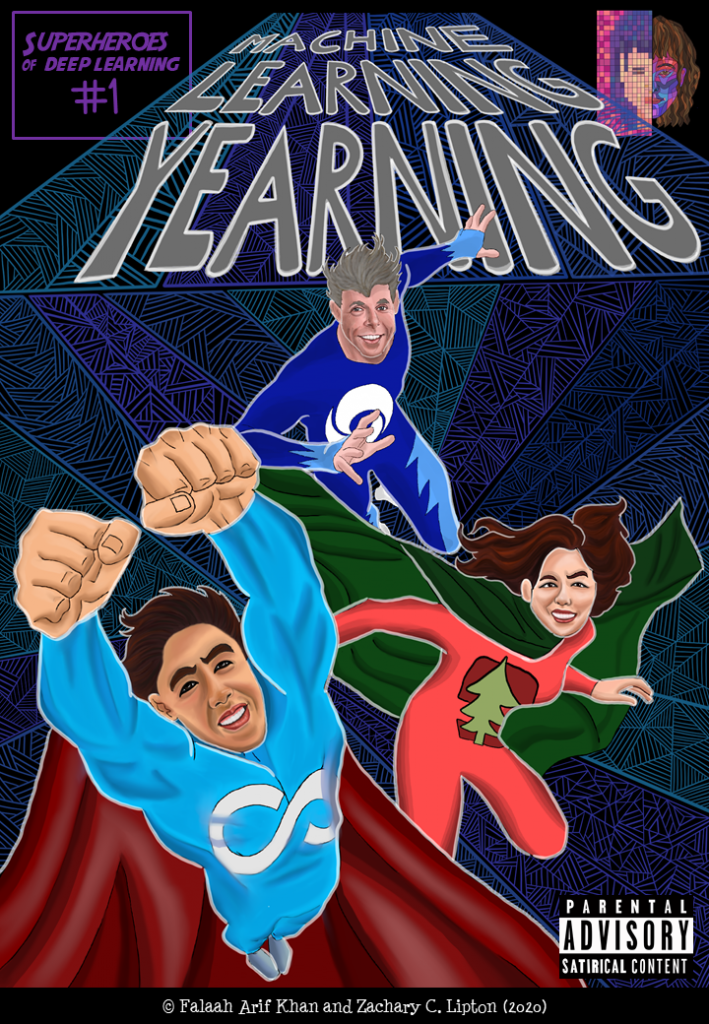
Technical and Social Perspectives on Machine Learning
Full PDFs free on GitHub. To support us, visit Patreon.
[This article originally appeared on the Deep Safety blog.]
This year’s NIPS gave me a general sense that near-term AI safety is now mainstream and long-term safety is slowly going mainstream. On the near-term side, I particularly enjoyed Kate Crawford’s keynote on neglected problems in AI fairness, the ML security workshops, and the Interpretable ML symposium debate that addressed the “do we even need interpretability?” question in a somewhat sloppy but entertaining way. There was a lot of great content on the long-term side, including several oral / spotlight presentations and the Aligned AI workshop.
[This article is a revised version reposted with permission from KDnuggets]
Imagine you’re a doctor tasked with choosing a cancer therapy. Or a Netflix exec tasked with recommending movies. You have a choice. You could think hard about the problem and come up with some rules. But these rules would be overly simplistic, not personalized to the patient or customer. Alternatively, you could let the data decide what to do!
The ability to programmatically make intelligent decisions by learning complex decision rules from big data is a driving selling point of machine learning. Leaps forward in the predictive accuracy of supervised learning techniques, especially deep learning, now yield classifiers that outperform human predictive accuracy on many tasks. We can guess how an individual will rate a movie, classify images, or recognize speech with jaw-dropping accuracy. So why not make our services smart by letting the data tell us what to do?
Continue reading “The Deception of Supervised Learning – V2”