Full PDFs free on GitHub. To support us, visit Patreon.
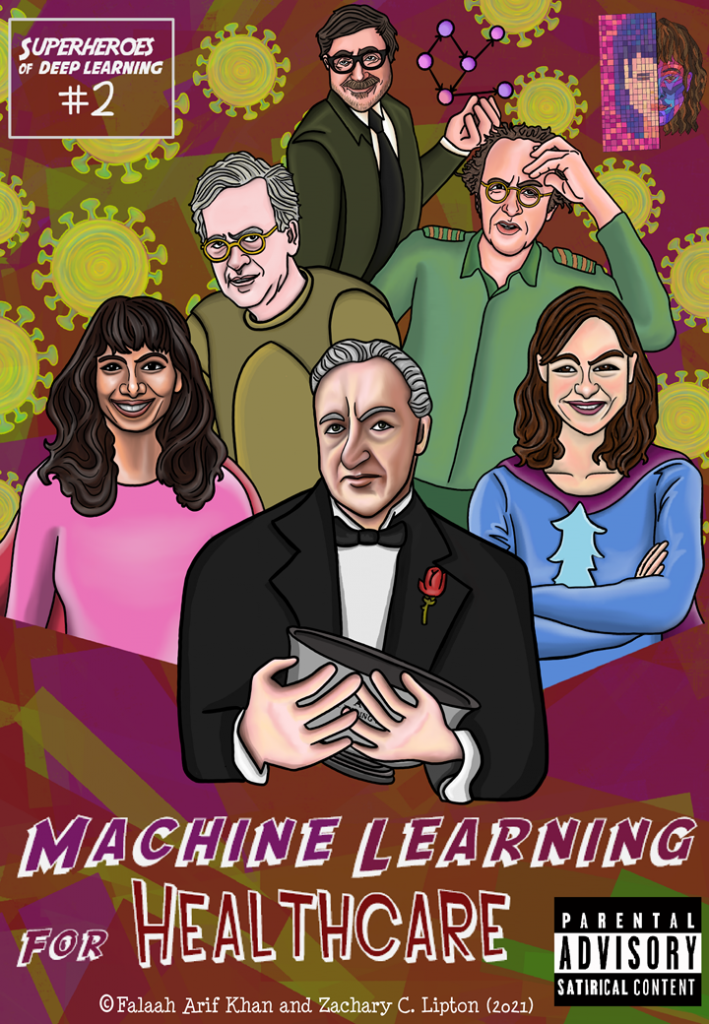
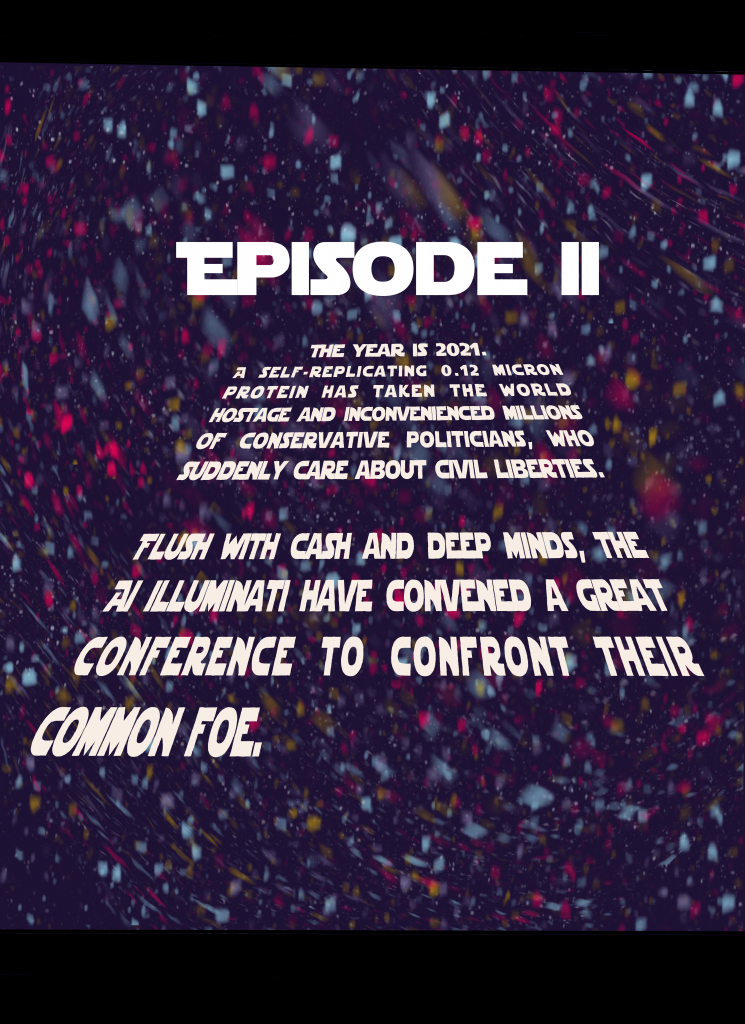
![Meanwhile spurned by the ML elite, DAG-man de-stresses on a California beach with some sunnies and funnies…
[DAG-Man] On beach, lounge chair, sipping beach cocktail with little umbrella & pineapple slice,
Reading DL Superheroes Vol 1.
[Tweeting out on Blackberry --- “Translation please? I grew up with Popeye and Little Red Riding Hood....” ]](https://www.approximatelycorrect.com/wp-content/uploads/2021/08/2-745x1024.png)
![[Somewhere in Westchester county...]
[Giant mansion, inspired by Prof X’s school for the gifted—long trail of mafia town cars lined up outside. At the entrance is a long line and a registration desk, with 1000s of people in line to get their badge and nametag]
[Poster outside says International Conference for ML Superheroes 2021]
[All the factions of the ML community are here, The DL Superheroes, Rigor Police (dressed up like British bobbies), The Algorithmic Justice League, The Causal Conspirators, and the Symbol Slappers ]](https://www.approximatelycorrect.com/wp-content/uploads/2021/08/3-745x1024.png)
![Anon char 1: How did the Superheroes afford this place?
Anon char 2: Was the Element AI acquihire more lucrative than we thought?
Anon char 3: ... I heard Captain Convolution got in early on Gamestop
Anon char 4: Shhh… he’s about to speak...
The GodFather: I look around, I look around,
and I see a lot of familiar faces.
[nods at each]
Don Valiant, Donna Boulamwini, GANfather....
It’s not every day that we gather
the entire family under one roof.
We unite here today
And put aside our differences
because a gathering threat
imperils our common interests
You may already know...](https://www.approximatelycorrect.com/wp-content/uploads/2021/08/4-745x1024.png)
![[Tensorial Professor]
The curse of dimensionality?
[Kernel Scholkopf]
Confounding?
[Code Poet]
Injustice?
[The GANfather]
Schmidhubering?](https://www.approximatelycorrect.com/wp-content/uploads/2021/08/6-1-745x1024.png)
Technical and Social Perspectives on Machine Learning
Full PDFs free on GitHub. To support us, visit Patreon.
Authors: Liu Leqi, Dylan Hadfield-Menell, and Zachary C. Lipton
To appear in Communications of the ACM (CACM) and available on arXiv.org.
Ever since social activity on the Internet began migrating from the wilds of the open web to the walled gardens erected by so-called platforms (think Myspace, Facebook, Twitter, YouTube, or TikTok), debates have raged about the responsibilities that these platforms ought to bear. And yet, despite intense scrutiny from the news media and grassroots movements of outraged users, platforms continue to operate, from a legal standpoint, on the friendliest terms.
You might say that today’s platforms enjoy a “have your cake, eat it too, and here’s a side of ice cream” deal. They simultaneously benefit from: (1) broad discretion to organize (and censor) content however they choose; (2) powerful algorithms for curating a practically limitless supply of user-posted microcontent according to whatever ends they wish; and (3) absolution from almost any liability associated with that content.
This favorable regulatory environment results from the current legal framework, which distinguishes between intermediaries (e.g., platforms) and content providers. This distinction is ill-adapted to the modern social media landscape, where platforms deploy powerful data-driven algorithms (so-called AI) to play an increasingly active role in shaping what people see and where users supply disconnected bits of raw content (tweets, photos, etc.) as fodder.
Continue reading “When Curation Becomes Creation”Full PDFs free on GitHub. To support us, visit Patreon.
While COVID has negatively impacted many sectors, bringing the global economy to its knees, one sector has not only survived but thrived: Data Science. If anything, the current pandemic has only scaled up demand for data scientists, as the world’s leaders scramble to make sense of the exponentially expanding data streams generated by the pandemic.
“These days the data scientist is king. But extracting true business value from data requires a unique combination of technical skills, mathematical know-how, storytelling, and intuition.” 1
Geoff Hinton
According to Gartner’s 2020 report on AI✝, 63% of the United States labor force has either (i) already transitioned; or (ii) is actively transitioning; towards a career in data science. However, the same report shows that only 5% of this cohort eventually lands their dream job in Data Science.
We interviewed top executives in Big Data, Machine Learning, Deep Learning, and Artificial General Intelligence; and distilled these 5 tips to guarantee success in Data Science.2
Continue reading “5 Habits of Highly Effective Data Scientists”Whether you are speaking to corporate managers, Silicon Valley script kiddies, or seasoned academics pitching commercial applications of their research, you’re likely to hear a lot of claims about what AI is going to do.
Hysterical discussions about AI machine learning’s applicability begin with a breathless recap of breakthroughs in predictive modeling (9X.XX% accuracy on ImageNet!, 5.XX% word error rate on speech recognition!) and then abruptly leap to prophesies of miraculous technologies that AI will drive in the near future: automated surgeons, human-level virtual assistants, robo-software development, AI-based legal services.
This sleight of hand elides a key question—when are accurate predictions sufficient for guiding actions?
By Zachary C. Lipton* & Jacob Steinhardt*
*equal authorship
Originally presented at ICML 2018: Machine Learning Debates [arXiv link]
Published in Communications of the ACM
Collectively, machine learning (ML) researchers are engaged in the creation and dissemination of knowledge about data-driven algorithms. In a given paper, researchers might aspire to any subset of the following goals, among others: to theoretically characterize what is learnable, to obtain understanding through empirically rigorous experiments, or to build a working system that has high predictive accuracy. While determining which knowledge warrants inquiry may be subjective, once the topic is fixed, papers are most valuable to the community when they act in service of the reader, creating foundational knowledge and communicating as clearly as possible.
What sort of papers best serve their readers? We can enumerate desirable characteristics: these papers should (i) provide intuition to aid the reader’s understanding, but clearly distinguish it from stronger conclusions supported by evidence; (ii) describe empirical investigations that consider and rule out alternative hypotheses [62]; (iii) make clear the relationship between theoretical analysis and intuitive or empirical claims [64]; and (iv) use language to empower the reader, choosing terminology to avoid misleading or unproven connotations, collisions with other definitions, or conflation with other related but distinct concepts [56].
Recent progress in machine learning comes despite frequent departures from these ideals. In this paper, we focus on the following four patterns that appear to us to be trending in ML scholarship:
Last week, I flew from London to Tel Aviv. The man sitting to my right was a road warrior, just this side of a late-night bender in London. He was rocking an ostentatious pair of headphones and a pair of pants ripped wide apart at both knees. Perhaps a D.J.? At some point, circumstances emerged for us to commiserate over the experience of flying on Easyjet (not the easiest). Soon after, we stumbled through the obligatory airplane smalltalk: Where are you going? What do you do?
Turns out I was flying next to the CEO of an AI+Blockchain startup.
It’s always a bit surreal when I learn of entrepreneurs combining AI with blockchain technology. For the past few years, whenever I found my myself bored among Silicon Valley socialites, this was my go-to satirical startup. What do you do? Startup CEO. What does your startup do? Deep learning on the blockchain… in The Cloud. Whoa. Continue reading “The Blockchain Bubble will Pop, What Next?”
Artificial intelligence is transforming the way we work (Venture Beat), turning all of us into hyper-productive business centaurs (The Next Web). Artificial intelligence will merge with human brains to transform the way we think (The Verge). Artificial intelligence is the new electricity (Andrew Ng). Within five years, artificial intelligence will be behind your every decision (Ginni Rometty of IBM via Computer World ).
Before committing all future posts to the coming revolution, or abandoning the blog altogether to beseech good favor from our AI overlords at the AI church, perhaps we should ask, why are today’s headlines, startups and even academic institutions suddenly all embracing the term artificial intelligence (AI)?
In this blog post, I hope to prod all stakeholders (researchers, entrepreneurs, venture capitalists, journalists, think-fluencers, and casual observers alike) to ask the following questions:
In 2014, Szegedy et al. published an ICLR paper with a surprising discovery: modern deep neural networks trained for image classification exhibit the following vulnerability: by making only slight alterations to an input image, it’s possible to drastically fool a model that would otherwise classify the image correctly (say, as a dog), into outputting a completely wrong label (say, as a banana). Moreover, this attack is possible even with perturbations that are so tiny that a human couldn’t distinguish the altered image from the original.
These doctored images are called adversarial examples and the study of how to make neural networks robust to these attacks is an increasingly active area of machine learning research.
Continue reading “Leveraging GANs to combat adversarial examples”
It’s January 28th and I should be working on my paper submissions. So should you! But why write when we can meta-write? ICML deadlines loom only twelve days away. And KDD follows shortly after. The schedule hardly lets up there, with ACL, COLT, ECML, UAI, and NIPS all approaching before the summer break. Thousands of papers will be submitted to each.
The tremendous surge of interest in machine learning along with ML’s democratization due to open source software, YouTube coursework, and the availability of preprint articles are all exciting happenings. But every rose has a thorn. Of the thousands of papers that hit the arXiv in the coming month, many will be unreadable. Poor writing will damn some to rejection while others will fail to reach their potential impact. Even among accepted and influential papers, careless writing will sow confusion and damn some papers to later criticism for sloppy scholarship (you better hope Ali Rahimi and Ben Recht don’t win another test of time award!).
But wait, there’s hope! Your technical writing doesn’t have to stink. Over the course of my academic career, I’ve formed strong opinions about how to write a paper (as with all opinions, you may disagree). While one-liners can be trite, I learned early in my PhD from Charles Elkan that many important heuristics for scientific paper writing can be summed up in snappy maxims. These days, as I work with younger students, teaching them how to write clear scientific prose, I find myself repeating these one-liners, and occasionally inventing new ones.
The following list consists of easy-to-memorize dictates, each with a short explanation. Some address language, some address positioning, and others address aesthetics. Most are just heuristics so take each with a grain of salt, especially when they come into conflict. But if you’re going to violate one of them, have a good reason. This can be a living document, if you have some gems, please leave a comment.
Continue reading “Heuristics for Scientific Writing (a Machine Learning Perspective)”