Full PDFs free on GitHub. To support us, visit Patreon.
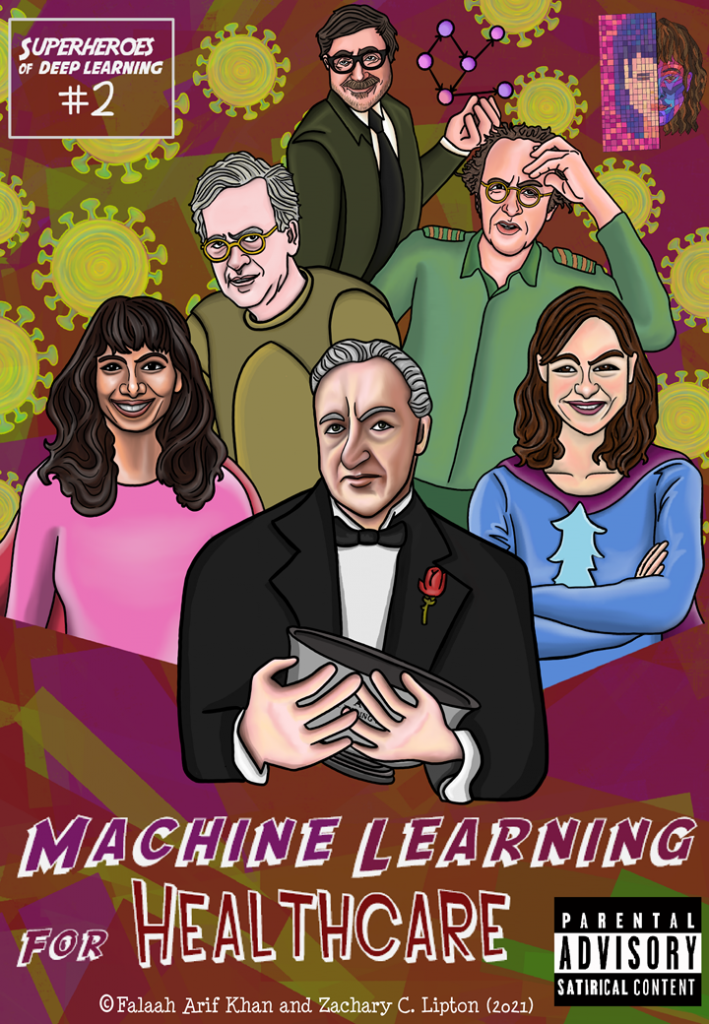
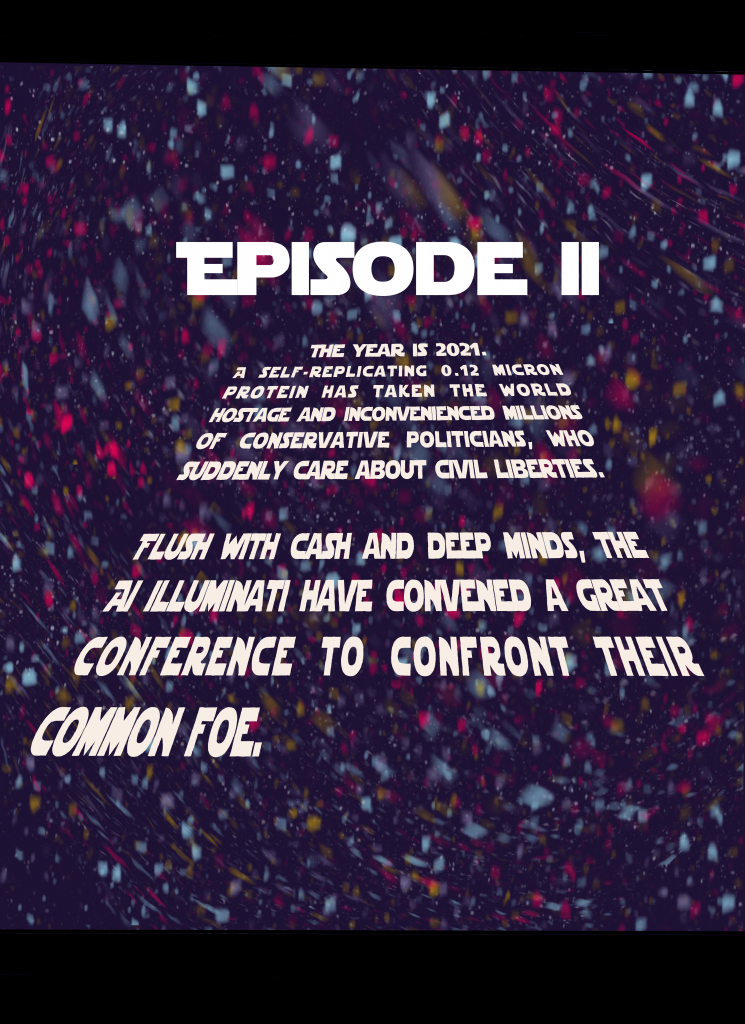
![Meanwhile spurned by the ML elite, DAG-man de-stresses on a California beach with some sunnies and funnies…
[DAG-Man] On beach, lounge chair, sipping beach cocktail with little umbrella & pineapple slice,
Reading DL Superheroes Vol 1.
[Tweeting out on Blackberry --- “Translation please? I grew up with Popeye and Little Red Riding Hood....” ]](https://www.approximatelycorrect.com/wp-content/uploads/2021/08/2-745x1024.png)
![[Somewhere in Westchester county...]
[Giant mansion, inspired by Prof X’s school for the gifted—long trail of mafia town cars lined up outside. At the entrance is a long line and a registration desk, with 1000s of people in line to get their badge and nametag]
[Poster outside says International Conference for ML Superheroes 2021]
[All the factions of the ML community are here, The DL Superheroes, Rigor Police (dressed up like British bobbies), The Algorithmic Justice League, The Causal Conspirators, and the Symbol Slappers ]](https://www.approximatelycorrect.com/wp-content/uploads/2021/08/3-745x1024.png)
![Anon char 1: How did the Superheroes afford this place?
Anon char 2: Was the Element AI acquihire more lucrative than we thought?
Anon char 3: ... I heard Captain Convolution got in early on Gamestop
Anon char 4: Shhh… he’s about to speak...
The GodFather: I look around, I look around,
and I see a lot of familiar faces.
[nods at each]
Don Valiant, Donna Boulamwini, GANfather....
It’s not every day that we gather
the entire family under one roof.
We unite here today
And put aside our differences
because a gathering threat
imperils our common interests
You may already know...](https://www.approximatelycorrect.com/wp-content/uploads/2021/08/4-745x1024.png)
![[Tensorial Professor]
The curse of dimensionality?
[Kernel Scholkopf]
Confounding?
[Code Poet]
Injustice?
[The GANfather]
Schmidhubering?](https://www.approximatelycorrect.com/wp-content/uploads/2021/08/6-1-745x1024.png)
Technical and Social Perspectives on Machine Learning
Full PDFs free on GitHub. To support us, visit Patreon.
While COVID has negatively impacted many sectors, bringing the global economy to its knees, one sector has not only survived but thrived: Data Science. If anything, the current pandemic has only scaled up demand for data scientists, as the world’s leaders scramble to make sense of the exponentially expanding data streams generated by the pandemic.
“These days the data scientist is king. But extracting true business value from data requires a unique combination of technical skills, mathematical know-how, storytelling, and intuition.” 1
Geoff Hinton
According to Gartner’s 2020 report on AI✝, 63% of the United States labor force has either (i) already transitioned; or (ii) is actively transitioning; towards a career in data science. However, the same report shows that only 5% of this cohort eventually lands their dream job in Data Science.
We interviewed top executives in Big Data, Machine Learning, Deep Learning, and Artificial General Intelligence; and distilled these 5 tips to guarantee success in Data Science.2
Continue reading “5 Habits of Highly Effective Data Scientists”What is a conference? Common definitions provide only a vague sketch: “a meeting of two or more persons for discussing matters of common concern” (Merriam Webster a); “a usually formal interchange of views” (Merriam-Webster b); “a formal meeting for discussion” (Google a).
What qualifies as a meeting? Are all congregations of people in all places conferences? How formal must it be? Must the borders be agreed upon? Does it require a designated name? What counts as a discussion? How many discussions can fit in one conference? Does a sufficiently formal meeting held within the allotted times and assigned premises of a larger, longer conference constitute a sub-conference?
Absent context, the word verges on vacuous. And yet in professional contexts, e.g., among computer science academics, culture endows precise meaning. Google also offers a more colloquial definitions that cuts closer:
Continue reading “The Greatest Trade Show North of Vegas (Pressing Lessons from NeurIPS 2018)”
By Zachary C. Lipton* & Jacob Steinhardt*
*equal authorship
Originally presented at ICML 2018: Machine Learning Debates [arXiv link]
Published in Communications of the ACM
Collectively, machine learning (ML) researchers are engaged in the creation and dissemination of knowledge about data-driven algorithms. In a given paper, researchers might aspire to any subset of the following goals, among others: to theoretically characterize what is learnable, to obtain understanding through empirically rigorous experiments, or to build a working system that has high predictive accuracy. While determining which knowledge warrants inquiry may be subjective, once the topic is fixed, papers are most valuable to the community when they act in service of the reader, creating foundational knowledge and communicating as clearly as possible.
What sort of papers best serve their readers? We can enumerate desirable characteristics: these papers should (i) provide intuition to aid the reader’s understanding, but clearly distinguish it from stronger conclusions supported by evidence; (ii) describe empirical investigations that consider and rule out alternative hypotheses [62]; (iii) make clear the relationship between theoretical analysis and intuitive or empirical claims [64]; and (iv) use language to empower the reader, choosing terminology to avoid misleading or unproven connotations, collisions with other definitions, or conflation with other related but distinct concepts [56].
Recent progress in machine learning comes despite frequent departures from these ideals. In this paper, we focus on the following four patterns that appear to us to be trending in ML scholarship:
With peak submission season for machine learning conferences just behind us, many in our community have peer-review on the mind. One especially hot topic is the arXiv preprint service. Computer scientists often post papers to arXiv in advance of formal publication to share their ideas and hasten their impact.
Despite the arXiv’s popularity, many authors are peeved, pricked, piqued, and provoked by requests from reviewers that they cite papers which are only published on the arXiv preprint.
“Do I really have to cite arXiv papers?”, they whine.
“Come on, they’re not even published!,” they exclaim.
The conversation is especially testy owing to the increased use (read misuse) of the arXiv by naifs. The preprint, like the conferences proper is awash in low-quality papers submitted by band-wagoners. Now that the tooling for deep learning has become so strong, it’s especially easy to clone a repo, run it on a new dataset, molest a few hyper-parameters, and start writing up a draft.
Of particular worry is the practice of flag-planting. That’s when researchers anticipate that an area will get hot. To avoid getting scooped / to be the first scoopers, authors might hastily throw an unfinished work on the arXiv to stake their territory: we were the first to work on X. All that follow must cite us. In a sublimely cantankerous rant on Medium, NLP/ML researcher Yoav Goldberg blasted the rising use of the (mal)practice. Continue reading “Do I really have to cite an arXiv paper?”
The following passage is a musing on the futility of futurism. While I present a perspective, I am not married to it.
When I sat down to write this post, I briefly forgot how to spell “dilemma”. Fortunately, Apple’s spell-check magnanimously corrected me. But it seems likely, if I were cast away on an island without any automatic spell-checkers or other people to subject my brain to the cold slap of reality, that my spelling would slowly deteriorate.
And just yesterday, I had a strong intuition about trajectories through weight-space taken by neural networks along an optimization path. For at least ten minutes, I was reasonably confident that a simple trick might substantially lower the number of updates (and thus the time) it takes to train a neural network.
But for the ability to test my idea against an unforgiving reality, I might have become convinced of its truth. I might have written a paper, entitled “NO Need to worry about long training times in neural networks” (see real-life inspiration for farcical clickbait title). Perhaps I might have founded SGD-Trick University, and schooled the next generation of big thinkers on how to optimize neural networks.
On Monday, I posted an article titled The AI Misinformation Epidemic. The article introduces a series of posts that will critically examine the various sources of misinformation underlying this AI hype cycle.
The post came about for the following reason: While I had contemplated the idea for weeks, I couldn’t choose which among the many factors to focus on and which to exclude. My solution was to break down the issue into several narrower posts. The AI Machine Learning Epidemic introduced the problem, sketched an outline for the series, and articulated some preliminary philosophical arguments.
To my surprise, it stirred up a frothy reaction. In a span of three days, the site received over 36,000 readers. To date, the article received 68 comments on the original post, 274 comments on hacker news, and 140 comments on machine learning subreddit.
To ensure that my post contributes as little novel misinformation as possible, I’d like to briefly address the response to the article and some common misconceptions shared by many comments. Continue reading “Notes on Response to “The AI Misinformation Epidemic””
This post introduces approximatelycorrect.com. The aspiration for this blog is to offer a critical perspective on machine learning. We intend to cover both technical issues and the fuzzier problems that emerge when machine learning intersects with society.
For explaining the technical details of machine learning, we enter a lively field. As recent breakthroughs in machine learning have attracted mainstream interest, many blogs have stepped up to provide high quality tutorial content. But at present, critical discussions on the broader effects of machine learning lag behind technical progress.
On one hand, this seems natural. First a technology must exist before it can have an effect. Consider the use of machine learning for face recognition. For many decades, the field has accumulated extensive empirical knowledge. But until recently, with the technology reduced to practice, any consideration of how it might be used could only be speculative.
But the precarious state of the critical discussion owes to more than chronology. It also owes to culture, and to the rarity of the relevant interdisciplinary expertise. The machine learning community traditionally investigates scientific questions. Papers address well-defined theoretical problems, or empirically compare methods with well-defined objectives. Unfortunately, many pressing issues at the intersection of machine learning and society do not admit such crisp formulations. But, with notable exceptions, consideration of social issues within the machine learning community remains too rare.
Conversely, those academics and journalists best equipped to consider economic and social issues rarely possess the requisite understanding of machine learning to anticipate the plausible ways the two arenas might intersect. As a result, coverage in the mainstream consistently misrepresents the state of research, misses many important problems, and hallucinates others. Too many articles address Terminator scenarios, overstating the near-term plausibility of human-like machine consciousness, assume the existence (at present) of self-motivated machines with their own desiderata, etc. Too few consider the precise ways that machine learning may amplify biases or perturb the job market.
In short, we see this troubling scenario:
Complicating matters, mainstream discussion of AI-related technologies introduces speculative or spurious ideas alongside sober ones without communicating uncertainty clearly. For example, the likelihood of a machine learning classifier making a mistake on a new example, and the likelihood of a machine learning causing massive unemployment and the likelihood of the entire universe being a simulation run by agents in some meta-universe are all discussed as though they can be a assigned some common form of probability.
Compounding the lack of rigor, we also observe a hype cycle in the media. PR machines actively promote sensational views of the work currently done in machine learning, even as the researchers doing that work view the hype with suspicion. The press also has an incentive to run with the hype: sensational news sells papers. The New York Times has referenced “Terminator” and “artificial intelligence” in the same story 5,000 times. It’s referenced “Terminator” and “machine learning” together in roughly 750 stories.
In this blog, we plan to bridge the gap between technical and critical discussions, treating both methodology and consequences as first-class concerns.
One aspiration of this blog will be to communicate honestly about certainty. We hope to maintain a sober, academic voice, even when writing informally or about issues that aren’t strictly technical. While many posts will express opinions, we aspire to clearly indicate which statements are theoretical facts, which may be speculative but reflect a consensus of experts, and which are wild thought experiments. We also plan to discuss both immediate issues such as employment alongside more speculative consideration of what future technology we might anticipate. In all cases we hope to clearly indicate scope. In service of this goal, and in reference to the theory of learning, we adopt the name Approximately Correct. We hope to be as correct as possible as often as possible, and to honestly convey our confidence.