Full PDFs free on GitHub. To support us, visit Patreon.
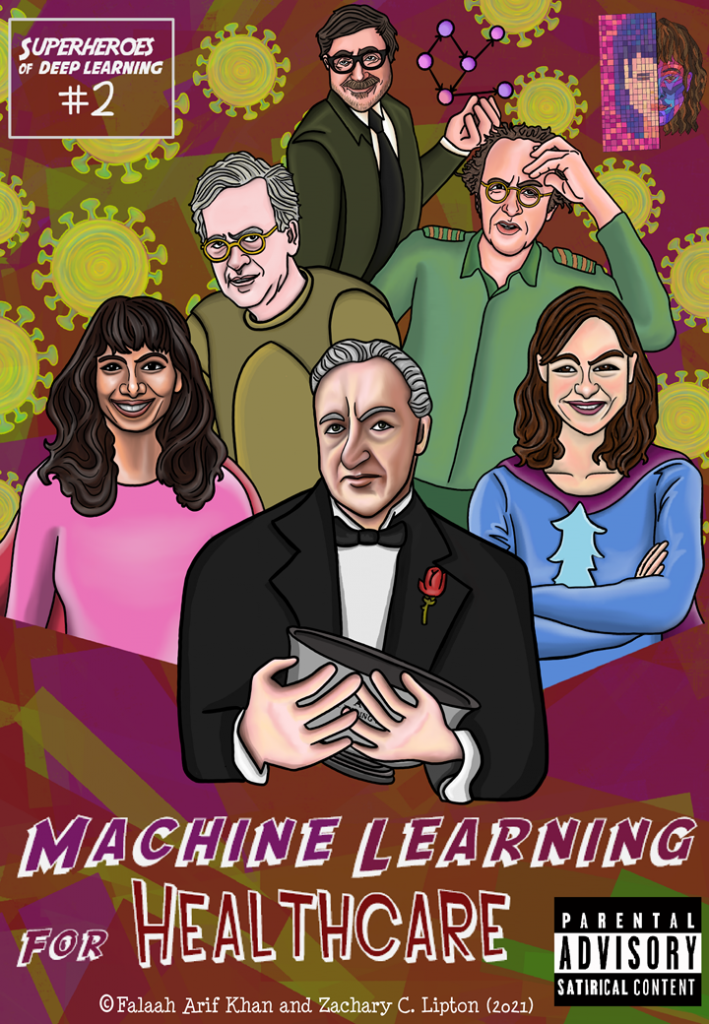
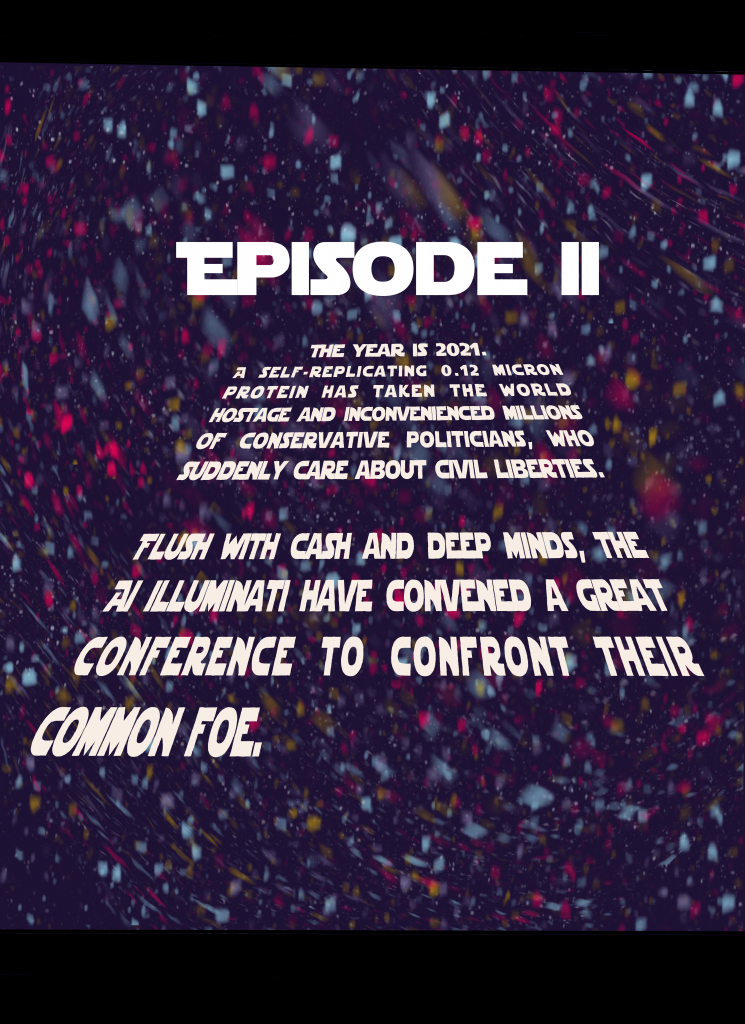
![Meanwhile spurned by the ML elite, DAG-man de-stresses on a California beach with some sunnies and funnies…
[DAG-Man] On beach, lounge chair, sipping beach cocktail with little umbrella & pineapple slice,
Reading DL Superheroes Vol 1.
[Tweeting out on Blackberry --- “Translation please? I grew up with Popeye and Little Red Riding Hood....” ]](https://www.approximatelycorrect.com/wp-content/uploads/2021/08/2-745x1024.png)
![[Somewhere in Westchester county...]
[Giant mansion, inspired by Prof X’s school for the gifted—long trail of mafia town cars lined up outside. At the entrance is a long line and a registration desk, with 1000s of people in line to get their badge and nametag]
[Poster outside says International Conference for ML Superheroes 2021]
[All the factions of the ML community are here, The DL Superheroes, Rigor Police (dressed up like British bobbies), The Algorithmic Justice League, The Causal Conspirators, and the Symbol Slappers ]](https://www.approximatelycorrect.com/wp-content/uploads/2021/08/3-745x1024.png)
![Anon char 1: How did the Superheroes afford this place?
Anon char 2: Was the Element AI acquihire more lucrative than we thought?
Anon char 3: ... I heard Captain Convolution got in early on Gamestop
Anon char 4: Shhh… he’s about to speak...
The GodFather: I look around, I look around,
and I see a lot of familiar faces.
[nods at each]
Don Valiant, Donna Boulamwini, GANfather....
It’s not every day that we gather
the entire family under one roof.
We unite here today
And put aside our differences
because a gathering threat
imperils our common interests
You may already know...](https://www.approximatelycorrect.com/wp-content/uploads/2021/08/4-745x1024.png)
![[Tensorial Professor]
The curse of dimensionality?
[Kernel Scholkopf]
Confounding?
[Code Poet]
Injustice?
[The GANfather]
Schmidhubering?](https://www.approximatelycorrect.com/wp-content/uploads/2021/08/6-1-745x1024.png)
Technical and Social Perspectives on Machine Learning
Full PDFs free on GitHub. To support us, visit Patreon.
Authors: Liu Leqi, Dylan Hadfield-Menell, and Zachary C. Lipton
To appear in Communications of the ACM (CACM) and available on arXiv.org.
Ever since social activity on the Internet began migrating from the wilds of the open web to the walled gardens erected by so-called platforms (think Myspace, Facebook, Twitter, YouTube, or TikTok), debates have raged about the responsibilities that these platforms ought to bear. And yet, despite intense scrutiny from the news media and grassroots movements of outraged users, platforms continue to operate, from a legal standpoint, on the friendliest terms.
You might say that today’s platforms enjoy a “have your cake, eat it too, and here’s a side of ice cream” deal. They simultaneously benefit from: (1) broad discretion to organize (and censor) content however they choose; (2) powerful algorithms for curating a practically limitless supply of user-posted microcontent according to whatever ends they wish; and (3) absolution from almost any liability associated with that content.
This favorable regulatory environment results from the current legal framework, which distinguishes between intermediaries (e.g., platforms) and content providers. This distinction is ill-adapted to the modern social media landscape, where platforms deploy powerful data-driven algorithms (so-called AI) to play an increasingly active role in shaping what people see and where users supply disconnected bits of raw content (tweets, photos, etc.) as fodder.
Continue reading “When Curation Becomes Creation”Full PDFs free on GitHub. To support us, visit Patreon.
If you’re not living under a rock, then you’ve surely encountered the Heroes of Deep Learning, an inspiring, diverse band of Deep Learning all-stars whose sheer grit, determination, and—[dare we say?]—genius, catalyzed the earth-shaking revolution that has brought to market such technological marvels as DeepFakes, GPT-7, and Gary Marcus.
But these are no ordinary times. And as the world contends with a rampaging virus, incendiary wildfires, and smouldering social unrest, no ordinary heroes will suffice. However, you needn’t fear. Hope has returned to the Machine Learning Universe, and boy, oh boy the timing couldn’t be better.
As confirmed to us by several independent witnesses, the sun, moon, and stars have been joined in the night’s sky by new, supernatural, sights. After a months-long meticulous investigation, including consultations with NASA, MI6, and Singularity University, we can confirm the presence, on Earth, of the Superheroes of Deep Learning!
Continue reading “Hope Returns to the Machine Learning Universe”While COVID has negatively impacted many sectors, bringing the global economy to its knees, one sector has not only survived but thrived: Data Science. If anything, the current pandemic has only scaled up demand for data scientists, as the world’s leaders scramble to make sense of the exponentially expanding data streams generated by the pandemic.
“These days the data scientist is king. But extracting true business value from data requires a unique combination of technical skills, mathematical know-how, storytelling, and intuition.” 1
Geoff Hinton
According to Gartner’s 2020 report on AI✝, 63% of the United States labor force has either (i) already transitioned; or (ii) is actively transitioning; towards a career in data science. However, the same report shows that only 5% of this cohort eventually lands their dream job in Data Science.
We interviewed top executives in Big Data, Machine Learning, Deep Learning, and Artificial General Intelligence; and distilled these 5 tips to guarantee success in Data Science.2
Continue reading “5 Habits of Highly Effective Data Scientists”On Thursday, OpenAI announced that they had trained a language model. They used a large training dataset and showed that the resulting model was useful for downstream tasks where training data is scarce. They announced the new model with a puffy press release, complete with this animation (below) featuring dancing text. They demonstrated that their model could produce realistic-looking text and warned that they would be keeping the dataset, code, and model weights private. The world promptly lost its mind.
For reference, language models assign probabilities to sequences of words. Typically, they express this probability via the chain rule as the product of probabilities of each word, conditioned on that word’s antecedents Alternatively, one could train a language model backwards, predicting each previous word given its successors. After training a language model, one typically either 1) uses it to generate text by iteratively decoding from left to right, or 2) fine-tunes it to some downstream supervised learning task.
Training large neural network language models and subsequently applying them to downstream tasks has become an all-consuming pursuit that describes a devouring share of the research in contemporary natural language processing.
Continue reading “OpenAI Trains Language Model, Mass Hysteria Ensues”
Whether you are speaking to corporate managers, Silicon Valley script kiddies, or seasoned academics pitching commercial applications of their research, you’re likely to hear a lot of claims about what AI is going to do.
Hysterical discussions about AI machine learning’s applicability begin with a breathless recap of breakthroughs in predictive modeling (9X.XX% accuracy on ImageNet!, 5.XX% word error rate on speech recognition!) and then abruptly leap to prophesies of miraculous technologies that AI will drive in the near future: automated surgeons, human-level virtual assistants, robo-software development, AI-based legal services.
This sleight of hand elides a key question—when are accurate predictions sufficient for guiding actions?
What is a conference? Common definitions provide only a vague sketch: “a meeting of two or more persons for discussing matters of common concern” (Merriam Webster a); “a usually formal interchange of views” (Merriam-Webster b); “a formal meeting for discussion” (Google a).
What qualifies as a meeting? Are all congregations of people in all places conferences? How formal must it be? Must the borders be agreed upon? Does it require a designated name? What counts as a discussion? How many discussions can fit in one conference? Does a sufficiently formal meeting held within the allotted times and assigned premises of a larger, longer conference constitute a sub-conference?
Absent context, the word verges on vacuous. And yet in professional contexts, e.g., among computer science academics, culture endows precise meaning. Google also offers a more colloquial definitions that cuts closer:
Continue reading “The Greatest Trade Show North of Vegas (Pressing Lessons from NeurIPS 2018)”
With paper submissions rocketing and the pool of experienced researchers stagnant, machine learning conferences, backs to the wall, have made the inevitable choice to inflate the ranks of peer reviewers, in the hopes that a fortified pool might handle the onslaught.
With nearly every professor and senior grad student already reviewing at capacity, conference organizers have gotten creative, finding reviewers in unlikely places. Reached for comment, ICLR’s program chairs declined to reveal their strategy for scouting out untapped reviewing talent, indicating that these trade secrets might be exploited by rivals NeurIPS and ICML. Fortunately, on condition of anonymity, several (less senior) ICLR officials agreed to discuss a few unusual sources they’ve tapped:
By Zachary C. Lipton* & Jacob Steinhardt*
*equal authorship
Originally presented at ICML 2018: Machine Learning Debates [arXiv link]
Published in Communications of the ACM
Collectively, machine learning (ML) researchers are engaged in the creation and dissemination of knowledge about data-driven algorithms. In a given paper, researchers might aspire to any subset of the following goals, among others: to theoretically characterize what is learnable, to obtain understanding through empirically rigorous experiments, or to build a working system that has high predictive accuracy. While determining which knowledge warrants inquiry may be subjective, once the topic is fixed, papers are most valuable to the community when they act in service of the reader, creating foundational knowledge and communicating as clearly as possible.
What sort of papers best serve their readers? We can enumerate desirable characteristics: these papers should (i) provide intuition to aid the reader’s understanding, but clearly distinguish it from stronger conclusions supported by evidence; (ii) describe empirical investigations that consider and rule out alternative hypotheses [62]; (iii) make clear the relationship between theoretical analysis and intuitive or empirical claims [64]; and (iv) use language to empower the reader, choosing terminology to avoid misleading or unproven connotations, collisions with other definitions, or conflation with other related but distinct concepts [56].
Recent progress in machine learning comes despite frequent departures from these ideals. In this paper, we focus on the following four patterns that appear to us to be trending in ML scholarship: